AI Choreography Rivals Human Creations
AI Choreography Rivals Human Creations
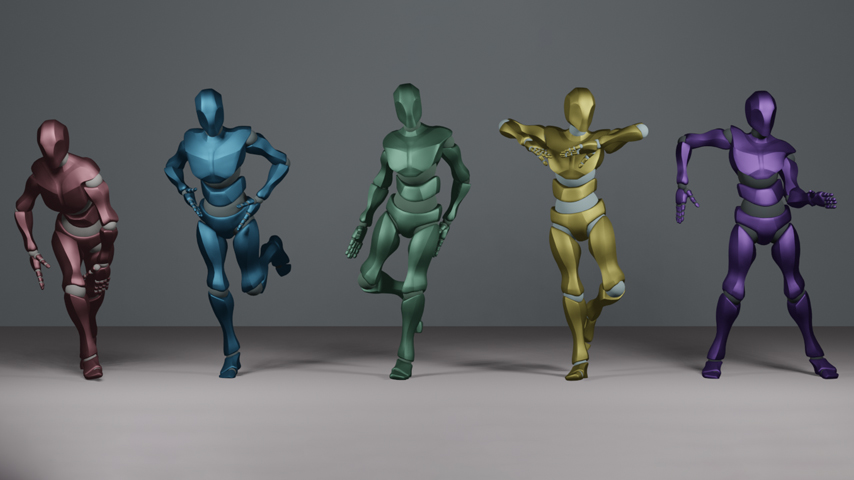
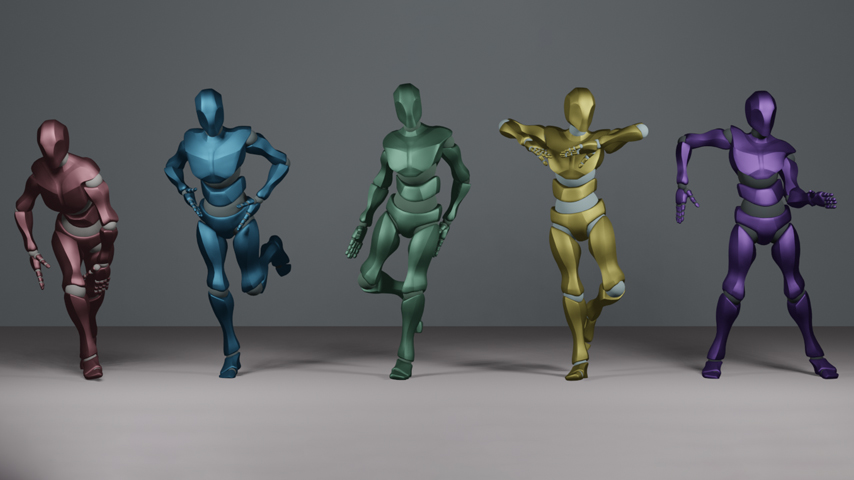
Stanford researchers have developed an AI tool that analyzes input music’s content and rhythms to create realistic dances.
The hottest up and coming choreographer just might be an AI tool called EDGE, short for Editable Dance GEneration. It’s already created dances that, according to the Stanford University research team behind it, study participants preferred over moves generated by other technologies. Or even, in some cases, moves designed by real humans.
“We do have requests from a dance company already” to use EDGE to choreograph a performance, said C. Karen Liu, associate professor of computer science at Stanford who led the team that includes student researchers Rodrigo Castellon, whose work focuses on the intersection of music and machine learning, and Jonathan Tseng, a computer science major who works on motion.
The funny thing is that Castellon, Tseng, and Liu are far from choreographers themselves. “Part of the reason we went into this project was because we’re so collectively bad at dancing,” Tseng joked, as the others concurred.
They may not be hoofers, but these scientists have contributed to la danse by continuing the “decades of work,” as Liu noted, in fields such as movement generation and computer graphics, and by building on previous machine-based learning methods and modern generation tools.
In their paper “EDGE: Editable Dance Generation From Music,” the team calls the project “a state-of-the-art method for dance generation that creates realistic, physically plausible dance motions based on input music.” The team uses “a transformer-based diffusion model paired with Jukebox,” a tool created by OpenAI that uses artificial intelligence trained on existing music to compose new music—like ChatGPT does with text.
In turn, EDGE trained its model on a dance motion dataset compiled from the motion capture of 39 dancers performing 10 genres: ballet, jazz, break, house, krump, LA-style hiphop, lock, middle hiphop, pop, street jazz, and waack. The dataset contains 10,108,015 frames of 3D keypoints with corresponding images and 1,408 human dance motion sequences, among other information.
The actual number of moves EDGE is capable of is more than these figures. “The dataset itself has 1,408 dance motions, i.e., clips of entire dances, but the model learns to mix and match these motions to produce new ones,” Castellon explained.
“In effect it looks like the model can do anything, but [it’s more like] the model can do what it’s done before,” Tseng said. Not copying, exactly, but as Liu puts it, “remeshing and resynthesizing” the moves.
Two of EDGE’s important capabilities are: “joint-wise conditioning,” in which the tool will generate upper body movements based on the lower body’s constraints, and vice versa; and “in-betweening,” which has two aspects, both of which can save a human choreographer hours of work.
In the first, a user can give start and end poses, and EDGE will generate dance sequences to fill the time between them. In the second, EDGE is given a seed motion, then told to continue for a length of time. “You can ask if, here’s some footwork, can you complete the dance?” Tseng said.
Despite the focus on technical methods, the team’s respect for the art is evident.
“Dance is not strictly about matching local minima in joint speed to beats. Instead, the beats of the music are a loose guide for timing, rhythm, and transitions between movements and dance steps,” the authors wrote in the academic paper when discussing how quantitative assessments used in similar past projects—like how exactly the movements align with the music’s beat—aren’t the best ways to evaluate an artistic form.
So, the researchers recruited a group of 147 human “raters,” sampled randomly from the population, to assess clips of dances that EDGE produced, and decide if they looked better or worse than dances that were either generated by other technologies or were choreographed by actual humans. Ultimately, the team says, more than 50 percent of the reviewers preferred EDGE’s creations.
The team presented EDGE in June at the Computer Vision and Pattern Recognition Conference in Vancouver, British Columbia. “We’re happy to say our project was well-received,” Castellon said. “Most visiting researchers mainly wanted to ask questions to understand our techniques better.”
Before the conference, Liu explained how EDGE could impact engineering.
“A lot of roboticists have expressed interest. We always want robots that can move like a human,” she said. “How can robots coexist with humans? That touches on a bigger picture that we focus on in the Movement Lab,” where Liu is director. The Stanford lab studies how to create, among other things, intelligent, natural, whole-body movements that real robots can use to interact with the world.
"We’re especially interested in human interaction, how robots interact, like if a robot has to touch a human [and] help someone off the bed or get into the car,” the professor said. EDGE can help scientists build a simulated world “that looks like our world; we can train robots in cheaper and safer ways."
Eydie Cubarrubia is an independent writer in New York.
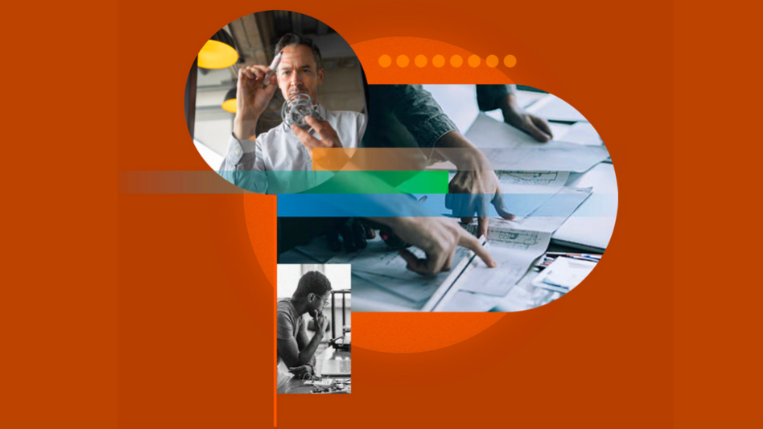